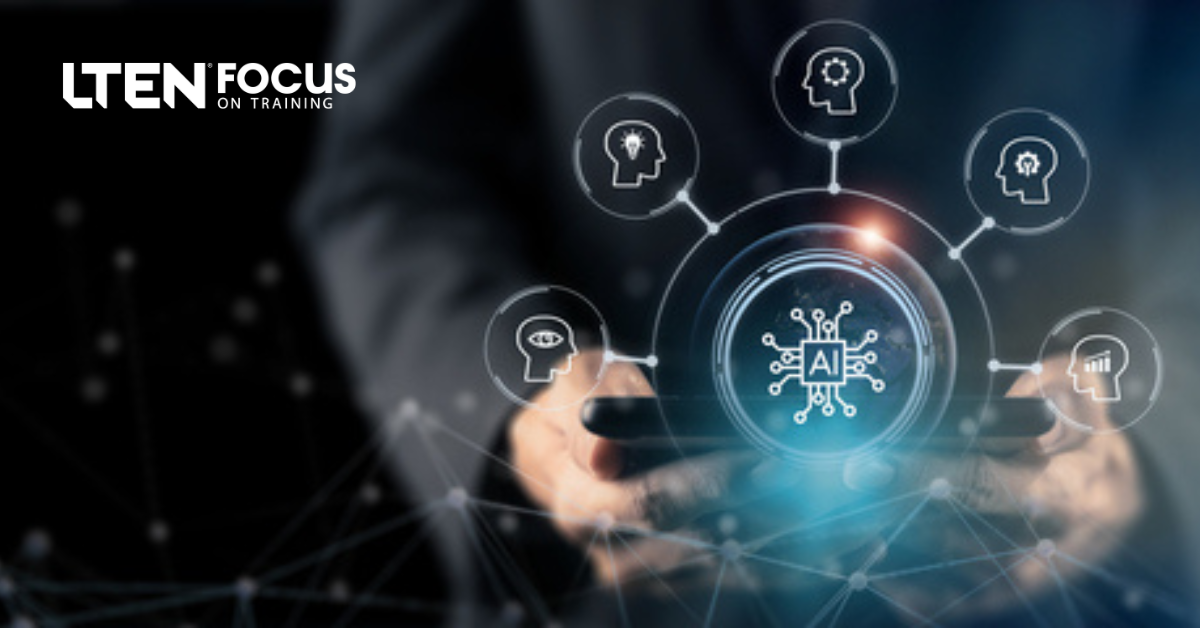
TRAINING TOOLS – By Brian Martin
Upskilling with AI is really just a reframing of communication
Have you ever engaged in the experiment of asking students to write directions on how to make a peanut butter and jelly sandwich? If you haven’t, it can be quite the entertaining spectacle to watch someone take someone else’s directions and try to build something edible.
The challenge of communicating knowledge and understanding between humans through language is a learned and practiced skill. It requires a frame of reference about common aspects of our human existence, cognition and perception.
How do you open a peanut butter jar? How do you remove bread from a bag? How do you determine the right proportion of peanut butter and jelly for a sandwich?
Level Up Your AI Skills
Everyone is on a journey with artificial intelligence. If you’re ready to learn more, plan to join us for #LTEN2024.
The final day of this year’s LTEN Annual Conference will focus exclusively on artificial intelligence in life sciences training. “Level Up AI: Transforming Ideas Into Action” will wrap up the event by exploring the transformative world of AI and its impact on life sciences training.
Find out more and register to join us at https://ltenconference.com/ai-day/.
We take many of these understandings of our environment and context for granted when we identify the person we’re writing the instructions for. The person is old enough to know the peanut butter jar lid opens by turning it counterclockwise. The person understands physical mechanisms enough to understand the plastic clip holding the bread bag closed is removed by levering one side of the clip’s tabs up and the other down. Everyone knows there should always be two parts peanut butter to one part jelly. (OK, maybe the proportions of PB to J is a potential hot button.)
With the advent of Generative AI (GenAI), and specifically GenAI as it applies to natural human languages, a new frontier of assistive communication technology is now at our disposal, with tools that can understand human languages in many ways more completely and accurately than we do. Tools that can process bodies of knowledge and extract insights at our direction faster and at greater scale than we as humans are able.
Wielding these tools through the power of the mystical new skill “prompt engineering” is a huge topic for those looking at upskilling and improving their capabilities. However, we need to set the record straight on prompt engineering.
Nothing Special and Nothing New
Every human being has been engaging in prompt engineering since the day they started communicating with language.
The way we communicate with other humans through language helps us to share knowledge and elicit a response from the other party. When we teach our children to say “please” and “thank you,” they are learning courtesy – but also learning that courteous engagement is more likely to elicit a positive response from the other party.
Failing to give someone complete instructions can result in an entire jar of peanut butter spread over an unopened bag of bread (I speak from experience). We automatically assume a certain level of knowledge and experience based on our perception of the recipient of our communication, and we engineer our communication – our prompt – to elicit the desired response.
From our first “I want a cookie” to yesterday’s “Will you please, please, please pickup your room,” we’ve been prompt engineering our entire lives.
Language Models: Eloquently Stupid
The large language models (LLM) that power the current GenAI wave today can be amazingly elegant, efficient and even exhilarating in their use of human languages. What they “know” is nothing more or less than how to predict the next word in a sentence – and they can do it with flair. Relying on the phrasing of the prompt and various parameters, they can output language that seems at times amazingly insightful.
However, don’t be confused – they know as much about quantum physics as Old Frank who sits at the end of the bar every night. They sure can sound authoritative, but fact-checking a straight LLM output is as necessary as parsing apolitical debate.
Facts matter, but not to an LLM; for an LLM, the only thing that matters is probabilities. This is an especially important consideration when you engineer a prompt for an LLM. Providing the appropriate context is essential, and sometimes providing that context needs to be paired with explicit instructions to only use the information provided in that context.
Rely on the LLM to interpret the language of your request and context, but require it to restrict itself to the facts and data provided. This is the heart of a process called retrieval augmented generation, or RAG in short.
More Science Than Engineering
The way to enhance any skill is to practice. While there may not be a state of perfection, regular experimentation will help build an understanding of the right way to communicate with the model.
Does the use of personas help deliver appropriately phrased results? Asking a model to respond as a specific persona can help it identify the content it has consumed that is likely to result in higher probability of a quality response.
Does breaking down the problem through chain-of-thought prompting deliver amore step-by-step reasoned output? Does giving examples help align the output more to the desired form of result? This is all a process of learning through experimentation – through which perception and understanding of the “mind” on the other end of the prompting is more well developed.
How we learn that telling a child they need to use the pointy end of the knife to spread the peanut butter is something we might never have anticipated having to say. But, there you have it (again, spoken from experience).
Conclusion
Ultimately, learning to take advantage of the power of LLM’s and GenAI’s potential in working with natural human languages is simply a matter of reframing communication.
Understand that it takes time and experimentation to get the right and desired outcomes. Don’t overestimate the power of a model as a source of knowledge, and be realistic in understanding how it actually works and what it can do well.
Embrace our power as human communicators to use nuance and clarity of direction to drive the tools to deliver optimal outputs. In the end, recognize that you’ve spent your whole life communicating knowledge and directing action to carbon-based lifeforms – now you’ve just got to figure out the same for silicon-based ones.
Oh, and consider having your kids say “please” and “thank you” when interacting with ChatGPT, Alexa or Google. Our future robot overlords may appreciate it.
Brian Martin is head of artificial intelligence for AbbVie Information Research and an AbbVie Research Fellow. Email Brian at brian.martin@abbvie.com or connect through linkedin.com/in/brianm1028.